Healthcare Ethical AI Lab (HEAL)
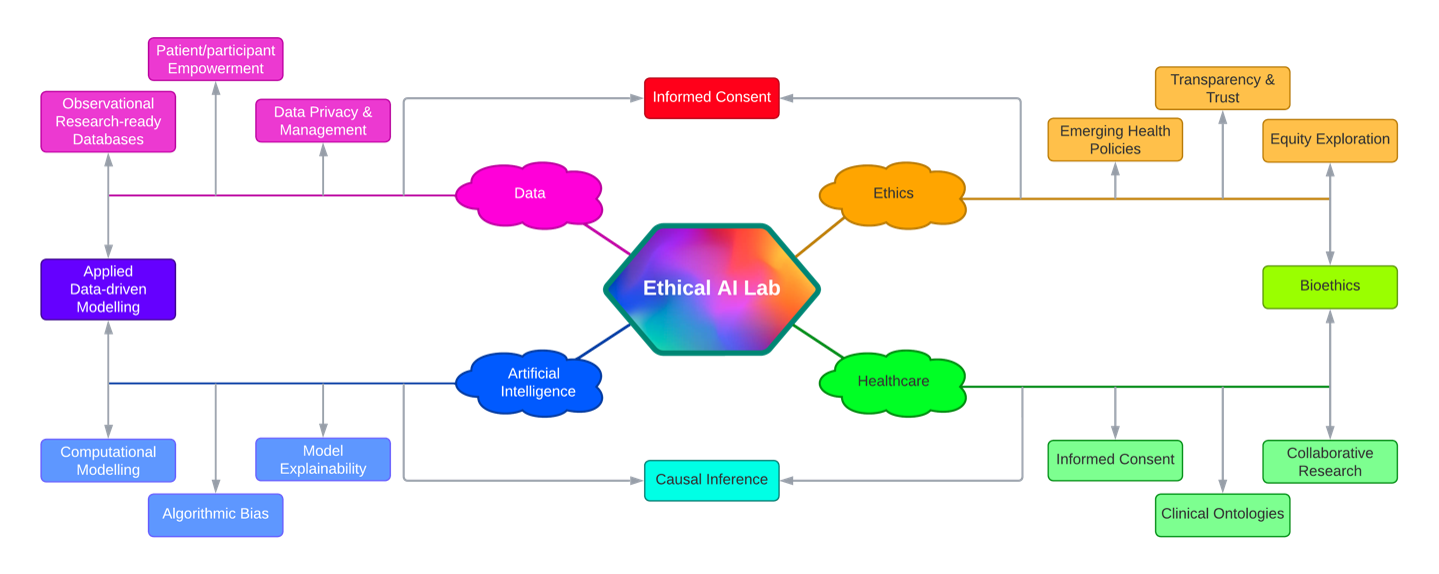
Summary
The Healthcare Ethical AI Lab (HEAL) is focused on the development and application of Transparent Algorithms and Ethical AI in Healthcare. Its research spans four main areas: data systems aligned with FAIR and CARE principles, development of ethical artificially intelligent systems, addressing healthcare challenges, and conducting analysis for integrated solutions focusing on health equity.
The research team, led by Mohammad Adibuzzaman, Ph.D., specializes in healthcare data curation, bioinformatics, and bioethics. This specialization supports the lab's goal of facilitating access to clinical data and enhancing the understanding of causal inference. The lab's research activities cover health equity, integration of multimodal data according to FAIR (Findability, Accessibility, Interoperability, Reusability) and CARE (Collective Benefit, Authority to Control, Responsibility, Ethics) principles, and the study of causal inference. These activities include both theoretical exploration and practical application, such as the evaluation of drug interventions with structural causal models.
In its approach to machine learning and artificial intelligence, the lab focuses on the use of interpretable models for Causal Inference to improve transparency in AI-driven decision-making processes, aiming to build trust between scientists, healthcare professionals and patients. Causal inference methodologies are utilized to identify relationships in healthcare outcomes, with counterfactual reasoning models supporting evidence-based decision-making. Efforts to address bias involve the implementation of strategies designed to prevent discriminatory outcomes in diverse patient populations, with a particular focus on the estimation and mitigation of bias.
The lab also emphasizes the importance of data privacy and security, developing data-sharing protocols that facilitate collaborative research while safeguarding patient information. Commitment to informed consent is key to the development of interactive tools designed to enhance patients' understanding of how their data is used and the implications of AI recommendations. Support for bioethics decision-making is integral, incorporating established guidelines and frameworks for ethical considerations in AI algorithms.
HEAL represents a collaborative research environment that combines the expertise of clinicians and computer scientists to promote ethical practices in the application of AI within healthcare.
Selected Publications and Projects
- Adibuzzaman, Mohammad, Poching DeLaurentis, Jennifer Hill, and Brian D. Benneyworth. "Big data in healthcare–the promises, challenges and opportunities from a research perspective: A case study with a model database." In AMIA Annual Symposium Proceedings, vol. 2017, p. 384. American Medical Informatics Association, 2017.
- Adibuzzaman, Mohammad, Ken Musselman, Alistair Johnson, Paul Brown, Zachary Pitluk, and Ananth Grama. "Closing the data loop: An integrated open access analysis platform for the mimic database." In 2016 Computing in Cardiology Conference (CinC), pp. 137-140. IEEE, 2016.
- Adibuzzaman, Mohammad, and Paul M. Griffin. "Big data in health care delivery." (2020).
- Gani, Md Osman, Shravan Kethireddy, Riddhiman Adib, Uzma Hasan, Paul Griffin, and Mohammad Adibuzzaman. "Structural causal model with expert augmented knowledge to estimate the effect of oxygen therapy on mortality in the icu." Artificial Intelligence in Medicine 137 (2023): 102493.
- Adib, Riddhiman, Md Osman Gani, Sheikh Iqbal Ahamed, and Mohammad Adibuzzaman. "Causal Discovery on the Effect of Antipsychotic Drugs on Delirium Patients in the ICU using Large EHR Dataset." arXiv preprint arXiv:2205.01057 (2022).
- Adib, Riddhiman, Paul Griffin, Sheikh Iqbal Ahamed, and Mohammad Adibuzzaman. "A causally formulated hazard ratio estimation through backdoor adjustment on structural causal model." In Machine Learning for Healthcare Conference, pp. 376-396. PMLR, 2020.
- Adib, Riddhiman, Md Mobasshir Arshed Naved, Chih-Hao Fang, Md Osman Gani, Ananth Grama, Paul Griffin, Sheikh Iqbal Ahamed, and Mohammad Adibuzzaman. "Ckh: Causal knowledge hierarchy for estimating structural causal models from data and priors." arXiv preprint arXiv:2204.13775 (2022).
- Adib, Riddhiman, Sheikh Iqbal Ahamed, and Mohammad Adibuzzaman. "Pragmatic Clinical Trials in the Rubric of Structural Causal Models." arXiv preprint arXiv:2204.13782 (2022).
- Chen, Yao, Xiao Wang, Yonghan Jung, Vida Abedi, Ramin Zand, Marvi Bikak, and Mohammad Adibuzzaman. "Classification of short single-lead electrocardiograms (ECGs) for atrial fibrillation detection using piecewise linear spline and XGBoost." Physiological measurement 39, no. 10 (2018): 104006.
- Bikak, M., R. Adib, W. Ingram, P. Griffin, and M. Adibuzzaman. "Outcomes of use of antipsychotic for delirium in the icu: A big data approach." In D50. CRITICAL CARE: THE METAMORPHOSIS-PAIN, SEDATION, DELIRIUM, ICU-ACQUIRED WEAKNESS, AND PALLIATIVE CARE, pp. A6672-A6672. American Thoracic Society, 2019.
- Fang, Chih-Hao, Vikram Ravindra, Salma Akhter, Mohammad Adibuzzaman, Paul Griffin, Shankar Subramaniam, and Ananth Grama. "Identifying and analyzing sepsis states: A retrospective study on patients with sepsis in ICUs." PLOS Digital Health 1, no. 11 (2022): e0000130.
- Patidar, Kavish R., Mobasshir A. Naved, Ananth Grama, Mohammad Adibuzzaman, Arzina Aziz Ali, James E. Slaven, Archita P. Desai et al. "Acute kidney disease is common and associated with poor outcomes in patients with cirrhosis and acute kidney injury." Journal of hepatology 77, no. 1 (2022): 108-115.
- Patidar, Kavish R., Mohammad Adibuzzaman, Mobasshir A. Naved, Dylan Rodriquez, James E. Slaven, Ananth Grama, Archita P. Desai et al. "Practice patterns and outcomes associated with intravenous albumin in patients with cirrhosis and acute kidney injury." Liver International 42, no. 1 (2022): 187-198.
Selected Active Projects
- Cyber-infrastructure for FAIR principle: Democratizing access to clinical data for research
- Democratizing access to clinical data for research: Implementation and evaluation strategies in an Academic Medical Center
- Radical Transparency for Healthcare Data: Policies, Technology and Implications
- Causal Discovery on the Effect of Antipsychotic Drugs on Delirium Patients in the ICU using Large Observational EHR Dataset
- Structural Causal Model with Expert Augmented Knowledge to Estimate the Effect of Oxygen Therapy on Mortality in the ICU
- Risk Stratification of Atrial Fibrillation for Patients with Ischemic Stroke: External Validation of the HAVOC Score
- Causal Knowledge Hierarchy for Estimating Structural Causal Models from Data with Priors in Clinical Research
- Backdoor adjustment of structural causal models in estimation of the Hazard ratio for survival analysis
- Tutorial on Fairness Analysis and Societal Bias Mitigation through Causal Inference
- Causal Inference for Rare Disease Cohort from N3C