Research
The Zuckerman Lab uses physics-based computational methods to study molecular, meso- and cell-scale systems. Starting from force laws for individual atoms, molecular simulation can provide a detailed picture of nanoscale biological behavior and aid rational drug design.
Molecular simulation, enhanced sampling, nonequilibrium statistical mechanics
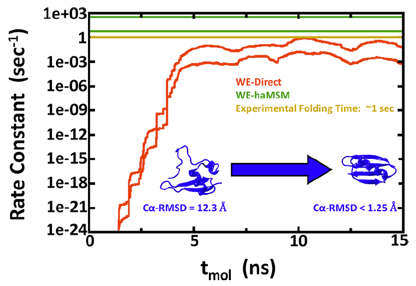
The group develops and applies simulation technology for studying mechanisms and kinetics in proteins and molecular machines. Our research addresses multiple challenges.
Algorithms: We develop physics-based enhanced molecular dynamics algorithms and software, largely focusing on the weighted ensemble (WE) method and WESTPA software. This work translates fundamental principles nonequilibrium statistical mechanics into practical algorithms and software.
Approximations: We leverage the theory of trajectories to develop Markov and non-Markov discrete-state approximations to continuous dynamical behavior with higher fidelity to molecular behavior than previously was possible.
Application: We apply these frameworks to study folding and conformational transitions in proteins, as well as ligand (un)binding processes. Allostery is another focus.
- Computational estimation of ms-sec atomistic folding times, Upendra Adhikari, Barmak Mostofian, Jeremy Copperman, Sundar Raman Subramanian, Andrew A. Petersen, and Daniel M. Zuckerman, J. Am. Chem. Soc., 141, 6519−6526 (2019).
- What Markov state models can and cannot do: Correlation versus path-based observables in protein folding models, Suarez, Ernesto; Wiewiora, Rafal; Wehmeyer, Christoph; Noé, Frank; Chodera, John; Zuckerman, Daniel, Journal of Chemical Theory and Computation, 17:3119–3133 (2021).
- Weighted ensemble: Recent mathematical developments, David Aristoff, Jeremy Copperman, Gideon Simpson, Robert Webber, and Daniel M. Zuckerman, J. Chem. Phys., 158, 014108 (2023).
Molecular machines and complexes – Bayesian inference
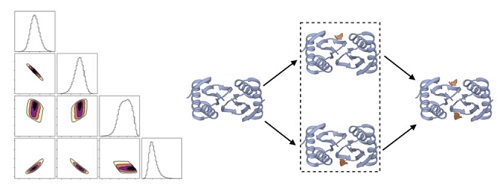
We study molecular complexes and machines using a variety of approaches, including image analysis, molecular simulation and Bayesian inference. We are particularly interested in the mechanisms by which machines function, which can be modeled via discrete states and Bayesian inference of experimental data.
- Vascular KATP channel structural dynamics reveal regulatory mechanism by Mg-nucleotides, Min Woo Sung, Zhongying Yang, Camden M. Driggers, Bruce L. Patton, Barmak Mostofian, John Russo, Daniel M. Zuckerman, & Show-Ling Shyng, Proceedings of the National Academy of Sciences, 118 (44) e2109441118 (2021).
- Continuum dynamics and statistical correction of compositional heterogeneity in multivalent IDP oligomers resolved by single-particle EM, Barmak Mostofian; Russell McFarland; Aidan Estelle; Jesse Howe; Elisar Barbar; Steve Reichow; Daniel M Zuckerman, J. Molec. Biol. 434: 167520 (2022).
- Quantifying cooperative multisite binding in the hub protein LC8 through Bayesian inference, Aidan B Estelle, August George, Elisar J Barbar, Daniel M Zuckerman, PLOS Comp. Biol. 19(4): e1011059 (2023).
Dynamical cell behavior – Morphology and omics
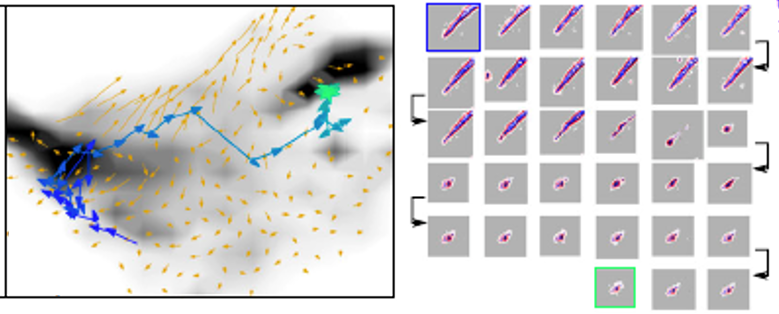
Despite our detailed understanding of myriad processes underpinning cell behavior, a true holistic and quantitative description has been lacking. We are attempting to integrate single-cell dynamical behavior captured in live-cell imaging with molecular readouts such as protein and RNA expression to develop a deeper understanding of cell biology. This project uses a combination of physics principles, machine learning and image analysis.
- Morphodynamical cell-state description via live-cell imaging trajectory embedding, Jeremy Copperman, Sean M. Gross, Young Hwan Chang, Laura M. Heiser, and Daniel M. Zuckerman, Comm. Biol., 6:484 (2023).